AI Essentials for In-House Lawyers: Tried-And-True Document Review Technologies
This Advisory aims to provide helpful insights regarding legal technology and artificial intelligence (AI) to in-house lawyers, particularly those with limited experience or knowledge in this area. Generative Artificial Intelligence (Gen AI) promises to be transformational in eDiscovery and has generated much hype and attention. However, these tools can be expensive and time consuming to deploy, and members of the bench and bar have expressed uncertainty about the technology’s present accuracy and effectiveness. Additionally, there is little developed case law on baseline requirements or best practices. It is thus important to remember that AI and other machine learning-powered and analytics-based tools, without generative features, have been part of the tech-savvy eDiscovery lawyer’s toolkit for many years and have made an enormous impact on the practice of law. In this Advisory we reiterate — and in some cases explain for the first time — that regardless of what Gen AI holds for the future of eDiscovery, attorneys today are already using remarkably effective and affordably priced AI tools and recommend that these tools not simply be discarded as new Gen AI tools are developed, tested, and improved.
Understanding AI Tools in eDiscovery
Gen AI differs from non-generative AI in that it creates new content, such as text or images. It takes in massive volumes of text, recognizes patterns from existing data, infers relationships among words, and applies what it has learned in order to generate new content consistent with the identified patterns and language relationships. Non-generative AI, on the other hand, analyzes data and performs tasks based on preexisting information and instruction. It uses algorithms to find patterns in data and make decisions based on those patterns. The key difference is that Gen AI generates new content, whereas traditional non-generative AI makes predictions or classifications.
AI tools that do not employ generative capabilities still significantly increase the speed and accuracy of an attorney’s work, while reducing client bills. Such AI tools are well understood, dependable, and effective. While looking toward the exciting future of Gen AI tools in the law, attorneys should not forget or underestimate the power of “ordinary” AI tools, and should consider their use, as appropriate, on client matters. Here are some of the tools we believe can be used in some way in nearly all eDiscovery matters:
Technology Assisted Review (TAR) and Continuous Active Learning (CAL) for Relevance Review
Attorneys have been using AI tools to enhance document review for relevance for years, with a significant impact upon the most cost-intensive part of litigation. Gone are the days of teams of contract attorneys poring over millions of documents for months or even years. Today, attorneys can utilize tools that leverage predictive coding techniques to identify relevant documents much faster, sometimes more accurately, and far more economically, than human reviewers do.
Such tools in their earliest form were referred to as Technology Assisted Review (TAR or TAR 1.0), and their use grew rapidly after 2012 with the endorsement of Magistrate Judge Andrew Peck in Da Silva Moore v. Publicis Groupe, 287 F.R.D. 182 (S.D.N.Y. 2012). TAR employs machine learning to help assess documents for relevance. First, human reviewers analyze a small set of randomly selected documents from the entire document collection for relevance. After rounds of additional sampling and review to refine predictive accuracy, the tool applies what it has learned from the small sample set to categorize the remaining documents, predicting which are relevant based on the patterns it has identified. In order to validate the results, the tool then generates a random, statistically significant selection of the documents categorized as not relevant, which are individually reviewed by humans to assess the effectiveness of the process.
Several years later, predictive coding developed into a more advanced method called Continuous Active Learning (CAL or TAR 2.0). In CAL, humans review a small percentage of documents and make relevance decisions on them one at a time, training the system to recognize patterns that it applies to the larger document universe. CAL continually refines its predictions by selecting small sets of new documents for human review, learning from each relevance decision. This ongoing process allows the system to prioritize documents likely to be relevant, enhancing accuracy and efficiency. Over time, using this iterative process, a corpus of relevant documents emerges. Finally, statistical analyses validate the model to show its effectiveness.
The knowledge a CAL system generates is not confined to a single data set; it can be applied across various cases and data sets for a client. For instance, an attorney might develop a CAL model to identify documents related to board meetings, which are often relevant in various litigations regardless of the subject matter. Once trained, this model can be reused in multiple litigations involving that client. This portability of the CAL model enables compounding efficiency gains by allowing the time invested in training to be amortized across cases with different data sets, eliminating the need to start from scratch with each new data set.
While perhaps not as exciting as Gen AI tools may prove to be, CAL is powerful and has saved attorneys countless hours and clients significant money. Attorneys should consider employing CAL (and, where appropriate, TAR) to increase efficiency and reduce legal spend on all but the smallest volume, most discrete, or highly specialized document reviews.
Concept Clustering
Concept clustering is a technology that helps organize large sets of documents by grouping them based on similar themes, and has several important applications. Significantly, concept clustering automatically sorts documents into visual, interactive data clusters without human review of the documents. The clusters provide a high-level overview of the document set so that attorneys can quickly gain critical insight into the kinds of documents that exist and the themes in the case without needing to review all of the underlying documents. Sometimes concept clustering will even reveal an otherwise hidden or non-intuitive connection or theme that might not be apparent when reviewing documents at the individual level. Attorneys can explore clusters and subclusters to develop themes and review particularly interesting documents in a cluster.
Attorneys use concept clustering to help find key documents that require immediate review, to set aside irrelevant clusters from the review pool, to find particularly relevant topics (and thus help develop search terms), and to perform quality control checks on groups of documents.
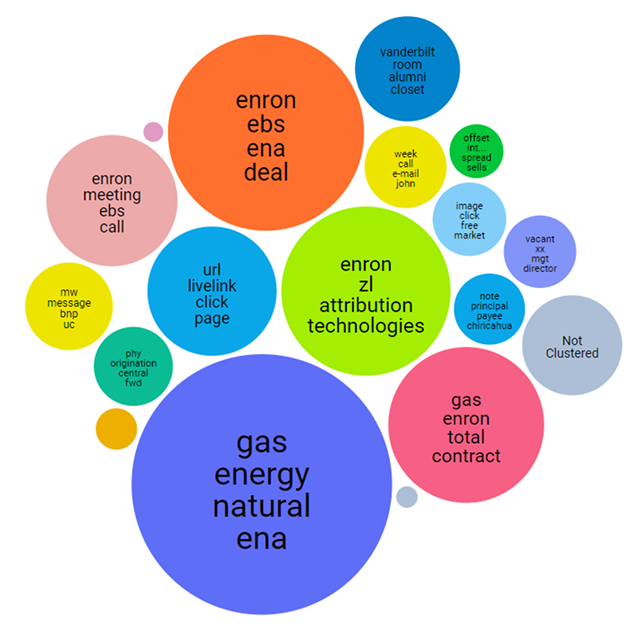
Attorneys can leverage concept clustering in other ways. For example, they can take key documents (from their client’s own collection or from opposing counsel’s documents) and use concept clustering techniques to quickly locate similar documents. Doing so early in the case can give attorneys an advantage over their adversaries and help develop their themes or defenses.
Relationship Analysis
Relationship analysis is an analytics tool to assist with examining patterns and connections within large sets of communications, like emails or messages (e.g., Slack, Teams). Before this technology existed, attorneys had to conduct custodial interviews to gain insights about important individuals in a case, including whom they interacted with. Attorneys could take information learned in the interviews to identify any additional custodians whose documents should be preserved and collected. Interviews are still extremely useful, but identifying all relevant custodians through interviews alone can be difficult and time consuming. Attorneys now use relationship analysis, which, when used in conjunction with traditional interviews and custodian questionnaires, can make the process much faster and more accurate earlier in the matter.
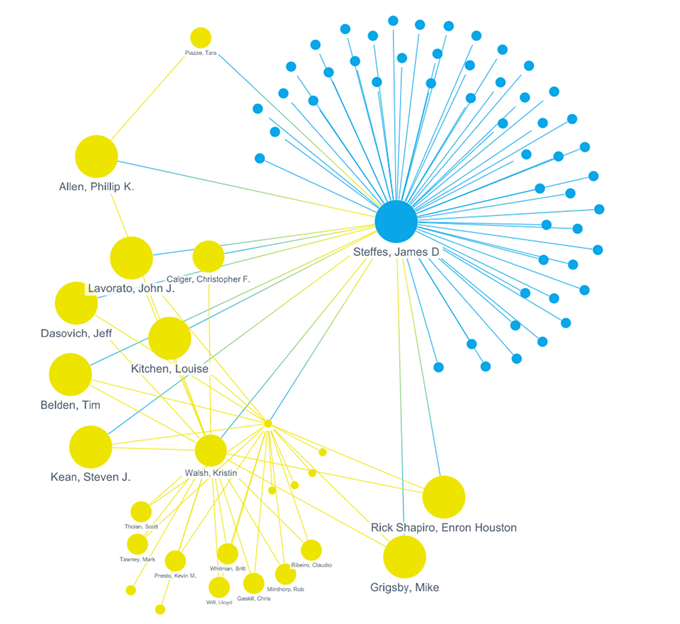
Relationship analysis automatically provides a visual illustration of communications among the various individuals within the data set. Reviewing these visualizations — but not all the underlying communications, which is cumbersome and time intensive — helps to confirm custodians and identify unexpected ones. Doing so early on can help attorneys with drafting initial disclosures and responding to discovery requests, as well as in their investigation and preservation efforts, and can lead to further, more refined fact development efforts.
Conclusion
This is just a sampling of the non-Gen AI-based tools readily available to attorneys, which continue to be powerful tools and valuable assets in the legal industry. There is no doubt that Gen AI is here to stay and will greatly expand the resources available to attorneys. However, employing new Gen AI tools in discovery and case development should not be done at the expense of the tried-and-true AI tools that have been proven to enhance case development and decrease litigation costs. In-house counsel, especially those with limited experience and knowledge in legal technology, should involve a tech-savvy lawyer early on in their matters to select the appropriate AI tools and employ them effectively on data sets. This will help ensure successfully litigating a matter at the lowest possible cost.
© Arnold & Porter Kaye Scholer LLP 2024 All Rights Reserved. This Advisory is intended to be a general summary of the law and does not constitute legal advice. You should consult with counsel to determine applicable legal requirements in a specific fact situation.